AI and Machine Learning Revolutionizes Post-Trade Risk Assessment
Discover the role of AI and machine learning in post-trade settlement. Learn about the benefits, challenges, and potential impact on the industry.
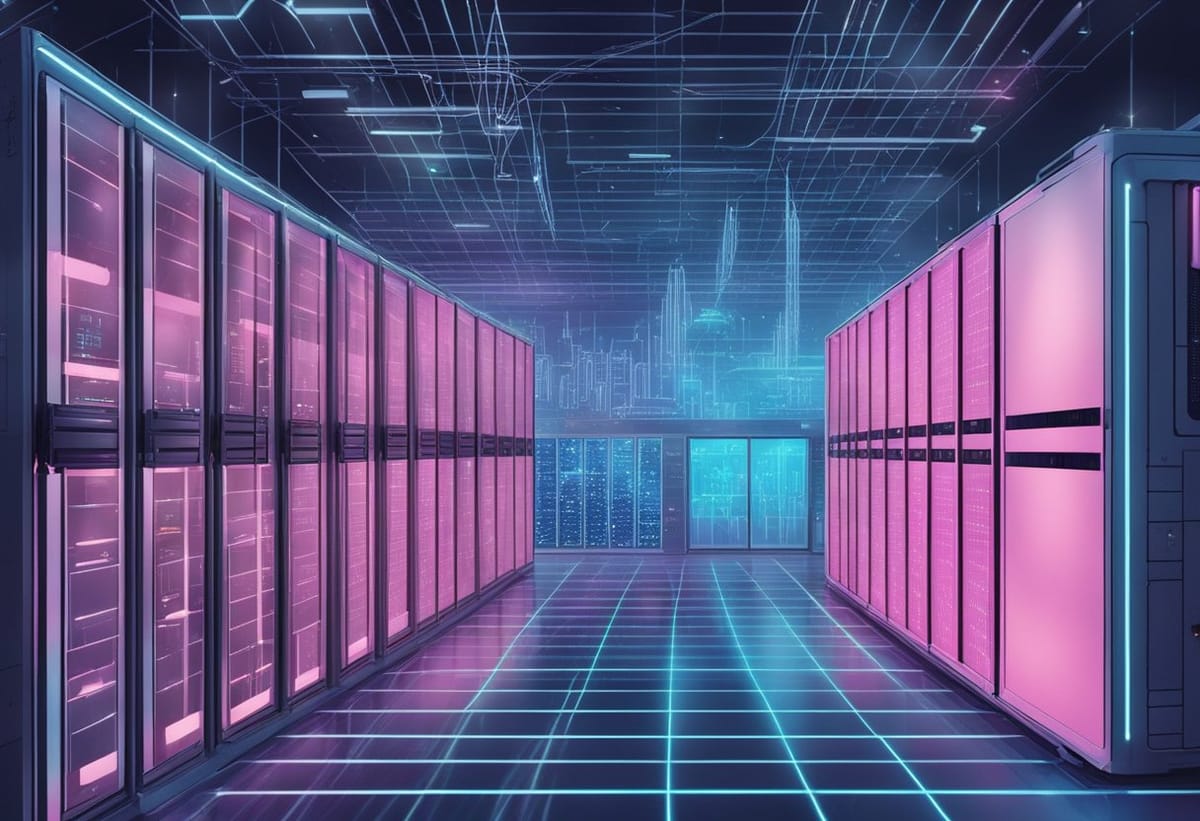
AI and machine learning have revolutionized the way businesses operate, especially in the financial sector. These technologies have enabled banks and other financial institutions to analyze vast amounts of data and make more informed decisions. Post-trade risk assessment is one area where AI and machine learning have been beneficial.
Post-trade risk assessment involves assessing the risk associated with financial transactions after they have been executed. This is an essential process as it helps financial institutions identify potential risks and take steps to mitigate them. AI and machine learning have made this process more efficient and effective by analyzing large volumes of data and identifying potential risks in real-time.
AI and machine learning used in post-trade risk assessment have become increasingly important in recent years as financial institutions face more complex and diverse risks. However, implementing these technologies can be challenging due to regulatory and compliance requirements. Despite these challenges, the benefits of using AI and machine learning in post-trade risk assessment are clear, and financial institutions are likely to continue to invest in these technologies to improve their risk management practices.
Key Takeaways
- AI and machine learning have revolutionized risk assessment in the financial sector.
- Post-trade risk assessment is a necessary process that helps financial institutions identify potential risks and take steps to mitigate them.
- Despite regulatory and compliance challenges, the benefits of using AI and machine learning in post-trade risk assessment are clear, and financial institutions are likely to continue to invest in these technologies to improve their risk management practices.
The Intersection of AI and Machine Learning
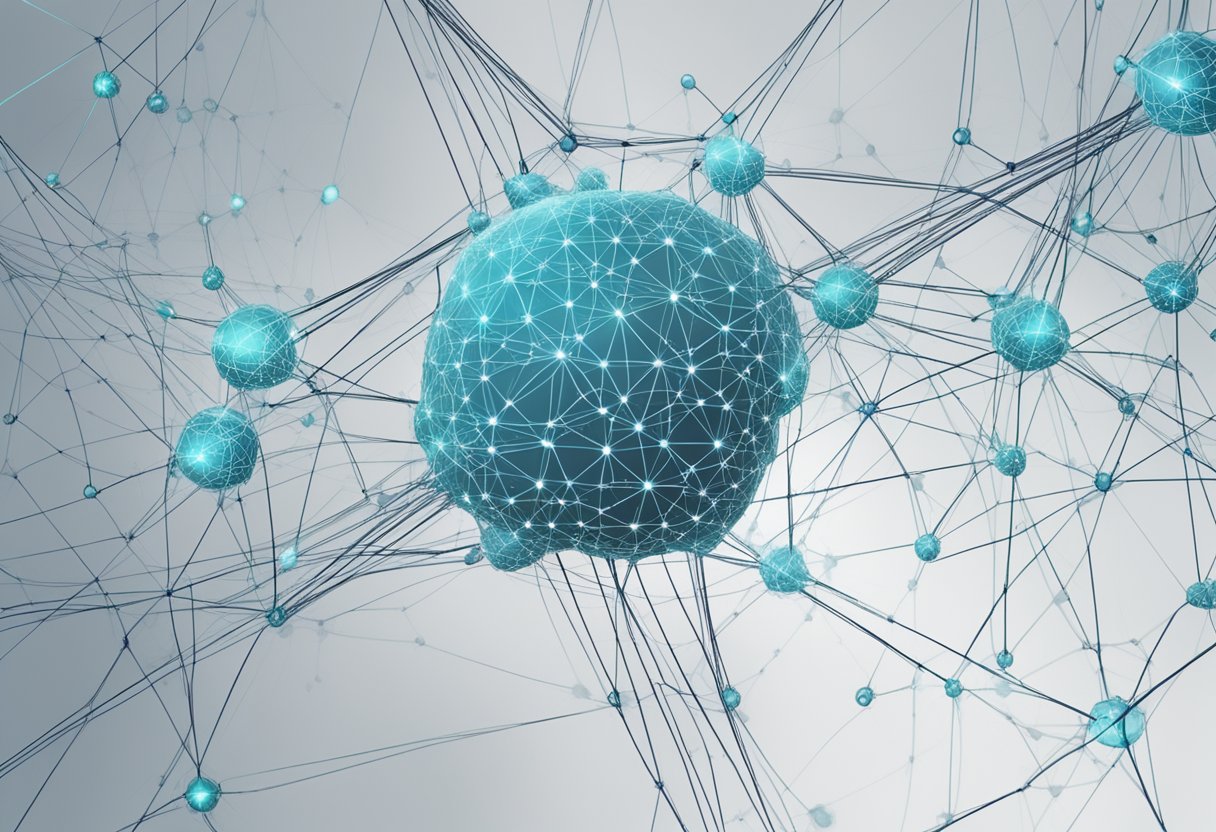
Differentiating AI and Machine Learning
Artificial Intelligence (AI) and Machine Learning (ML) are often used interchangeably but differ. AI refers to the ability of machines to perform tasks that typically require human intelligence, such as problem-solving, decision-making, and natural language processing. On the other hand, ML is a subset of AI that involves the use of algorithms to enable machines to learn from data and improve their performance over time.
The Role of AI and Machine Learning in Financial Services
The financial services industry has been an early adopter of AI and ML technologies, particularly in risk assessment. Post-trade risk assessment is a critical function in financial services, and AI and ML can help improve its efficiency and accuracy.
AI and ML can analyze large volumes of data, identify patterns and anomalies, and predict future market behaviour. This can help financial institutions identify potential risks and take appropriate actions to mitigate them. For example, ML algorithms can be used to analyze historical trade data and identify patterns that may indicate fraudulent activity.
AI and ML can also automate certain risk assessment aspects, such as data collection and analysis. This can help reduce the time and resources required for risk assessment and improve its accuracy and consistency.
Overall, the intersection of AI and ML has the potential to revolutionize post-trade risk assessment in financial services. However, it is essential to note that these technologies are not a silver bullet and must be used with human expertise and judgment.
Post-Trade Risk Assessment
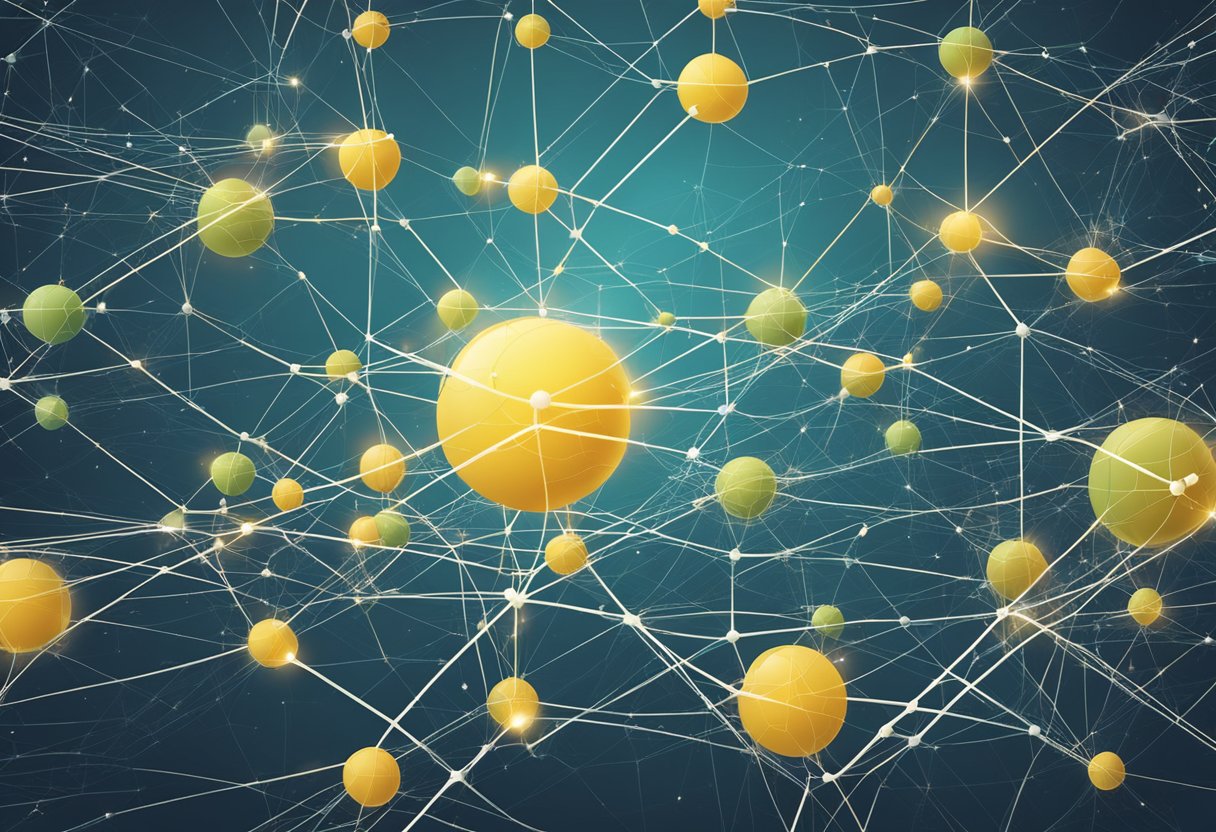
Post-trade processing is a critical function in capital markets firms. It involves confirming, clearing, and settling trades executed in financial markets. The process is complex and involves multiple parties, including brokers, custodians, clearinghouses, and settlement agents. Technology, particularly artificial intelligence (AI) and machine learning is transforming post-trade processing, making it more efficient, accurate, and secure.
Understanding Post-Trade Processes
Post-trade processes ensure that trades are confirmed, cleared, and settled accurately and efficiently. The confirmation process involves the exchange of trade details between counterparties to ensure that they agree on the terms of the trade. Clearing consists of the submission of trades to a clearinghouse, which acts as a central counterparty and guarantees the performance of the trade. Settlement consists of transferring cash and securities between the parties to the trade.
Post-trade processing is critical to the functioning of financial markets. It ensures that trades are settled promptly and accurately, reducing counterparty risk and promoting market stability. However, the process is complex and involves multiple parties, which can result in errors and delays. TI and machine learning can help streamline post-trade processing, making it more efficient and reducing the risk of errors.
The Importance of Risk Assessment in Post-Trade
Risk assessment is a critical function in post-trade processing. It involves identifying, measuring, and managing risks associated with trades. Risks can arise from a variety of sources, including market, credit, operational, and legal risks. TI and machine learning can help identify and manage risks associated with trades, making post-trade processing more efficient and secure.
AI and machine learning can help to automate risk assessment processes, making them faster and more accurate. Machine learning algorithms can analyze large volumes of data to identify patterns and anomalies that may indicate potential risks. They can also learn from historical data to improve their accuracy over time. AI can also help to automate risk management processes, making them more efficient and reducing the risk of errors.
In conclusion, AI and machine learning are transforming post-trade processing by making it more efficient, accurate, and secure. These technologies can help streamline post-trade processes, automate risk assessment and management, and reduce the risk of errors. As technology continues to evolve, it is likely that we will see further advancements in post-trade processing, which will continue to improve the functioning of financial markets.
AI and Machine Learning in Risk Management
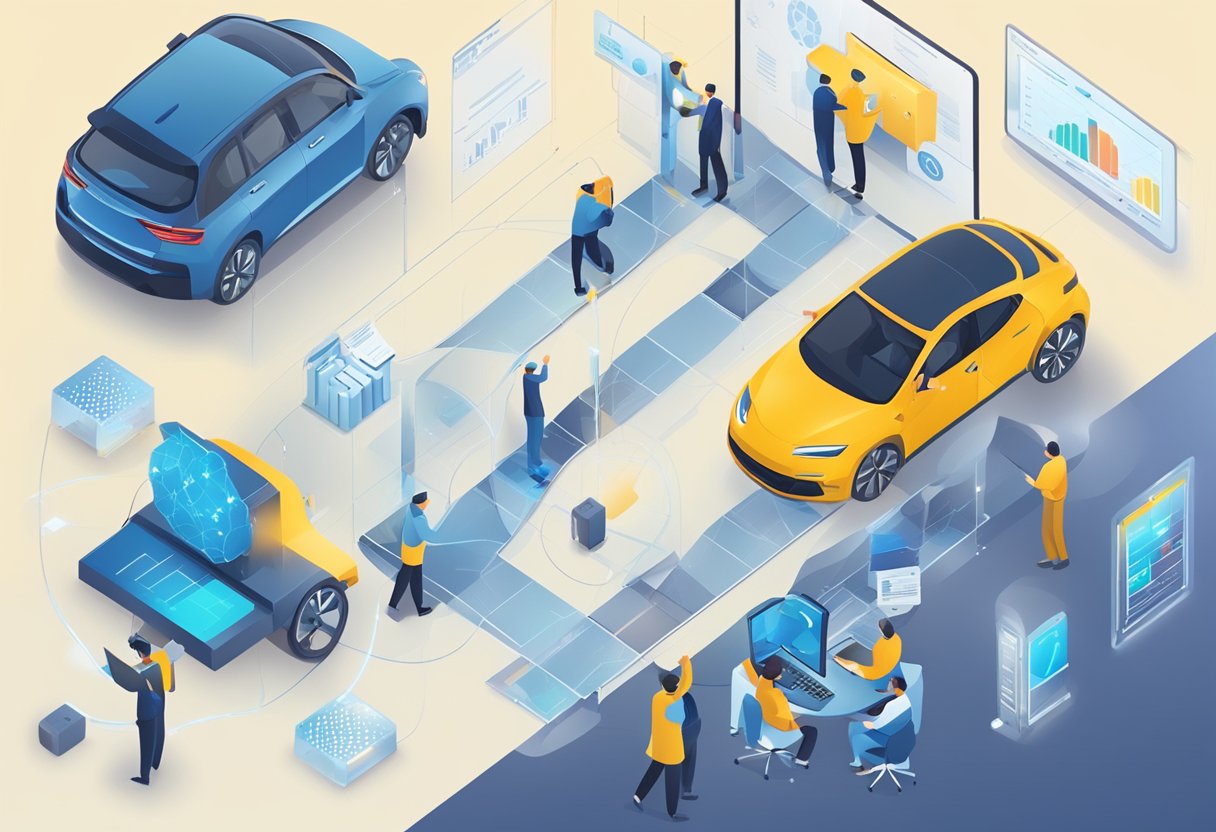
As the adoption of artificial intelligence (AI) and machine learning (ML) continues to grow, there is a growing interest in leveraging these technologies in risk management. AI and ML can help financial institutions automate processes, improve decision-making, and reduce operational costs. In post-trade risk assessment, AI and ML can help identify and mitigate risks associated with trades.
Leveraging AI in Risk Management
AI can be used to analyze large amounts of data to identify patterns and anomalies that may indicate risk. For example, AI algorithms can analyze trade data to identify potential fraud or market manipulation. AI can also be used to monitor market conditions and identify potential risks before they become a problem.
One of the benefits of AI is that it can learn from experience and adapt to changing market conditions. This means that AI can continuously improve its risk assessment capabilities over time. AI can also help automate routine tasks, freeing human resources to focus on more complex risk management tasks.
Machine Learning Models for Risk Assessment
Machine learning (ML) models can be used to predict future market conditions and identify potential risks. ML models can be trained on historical data to identify patterns and develop predictive models. These models can then be used to assess the risk associated with trades in real-time.
One of the benefits of ML is that it can identify patterns and relationships that may not be apparent to humans. This means that ML models can identify risks that traditional risk management methods may have missed. Additionally, ML models can help financial institutions make more informed decisions by providing real-time risk assessments.
However, it is essential to note that ML models are not foolproof. ML models can be susceptible to bias and may not always accurately predict future market conditions. Therefore, using ML models in conjunction with other risk management methods is essential to identify and address all potential risks.
In conclusion, AI and ML have the potential to revolutionize risk management in post-trade assessment. By leveraging these technologies, financial institutions can automate processes, improve decision-making, and reduce operational costs. However, using these technologies in conjunction with other risk management methods is essential to identify and address all potential risks.
The Impact of AI and Machine Learning on Compliance
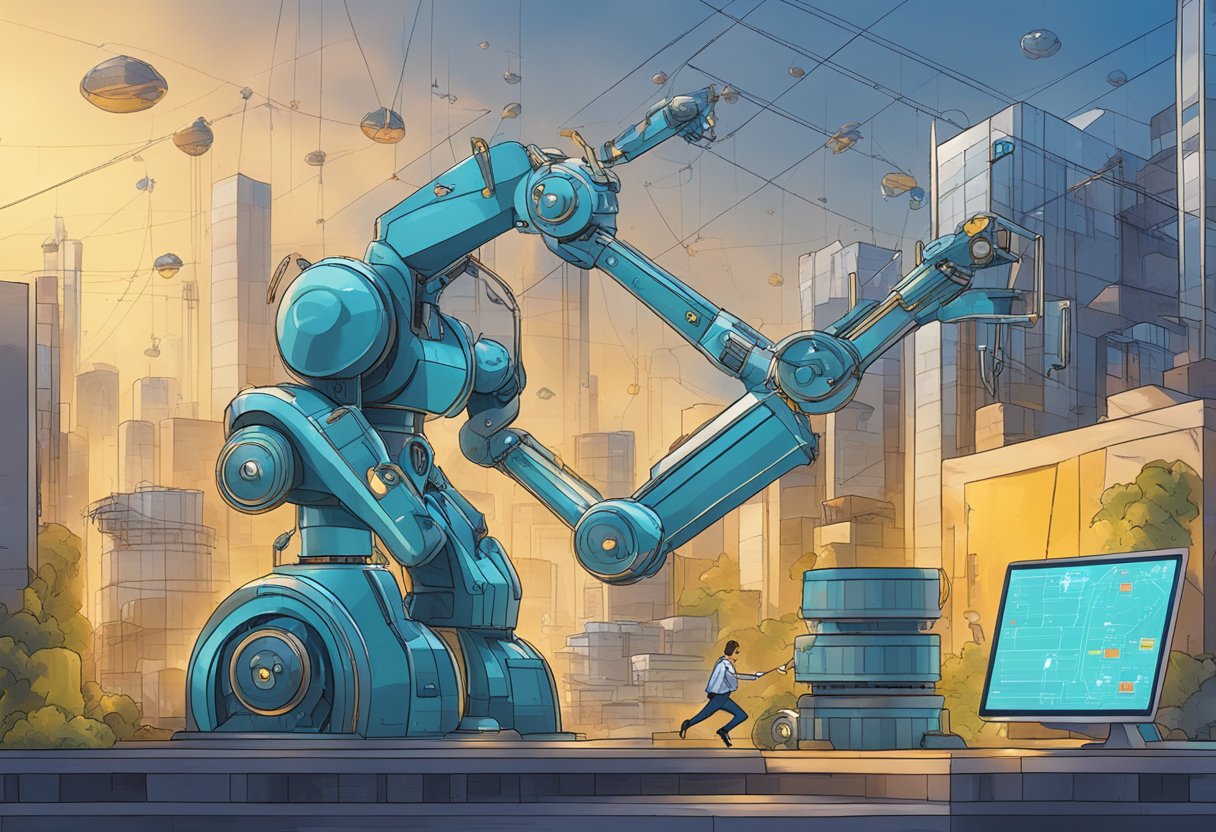
Artificial Intelligence (AI) and Machine Learning (ML) are revolutionizing the way financial institutions manage risk and compliance. By automating complex processes and analyzing vast amounts of data, AI and ML can help organizations identify and mitigate risks more effectively, reducing costs and increasing efficiency.
AI in Compliance Requirements
AI can play a crucial role in meeting compliance requirements by automating many of the processes involved in monitoring and reporting. For example, AI can help financial institutions identify and flag suspicious transactions more quickly and accurately than traditional methods. This can help prevent money laundering and other financial crimes, reducing the risk of regulatory fines and reputational damage.
In addition, AI can help organizations stay up-to-date with changing compliance requirements by analyzing regulatory updates and automatically updating policies and procedures accordingly. This can help organizations remain compliant while reducing the risk of human error.
Machine Learning in Anti-Money Laundering
Machine Learning (ML) is particularly useful in Anti-Money Laundering (AML) efforts, as it can help financial institutions identify patterns and anomalies in transaction data that may indicate money laundering or other financial crimes. ML algorithms can analyze vast amounts of data, including both structured and unstructured data, to identify suspicious activity more quickly and accurately than traditional methods.
ML can also help financial institutions improve risk assessments by analyzing data from various sources, including social media, news articles, and other public data. By analyzing this data, ML algorithms can identify potential risks and alert compliance teams to take action.
Overall, AI and ML are transforming the way financial institutions manage risk and compliance. By automating complex processes and analyzing vast amounts of data, these technologies can help organizations identify and mitigate risks more effectively, reducing costs and increasing efficiency.
AI and Machine Learning in Banking and Capital Markets
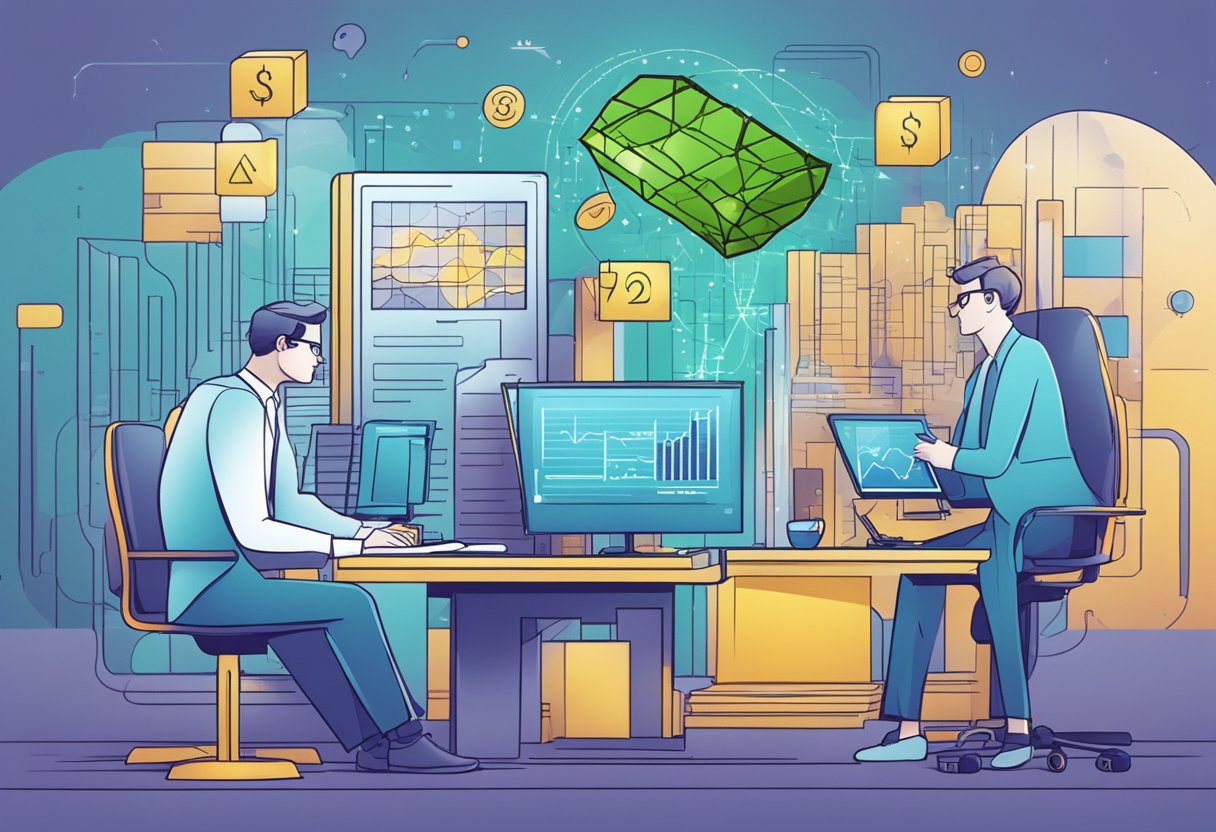
Artificial intelligence (AI) and machine learning (ML) have become integral to the banking and capital markets industry. These technologies improve operations, increase efficiency, and reduce costs. AI and ML are also used to enhance risk management by providing real-time market data analysis and identifying potential risks.
AI and Machine Learning in Banking Operations
In banking, AI and ML are being used to streamline operations and provide better customer service. These technologies are being used to automate customer service inquiries, fraud detection, and loan underwriting tasks. AI and ML are also being used to provide personalized recommendations to customers based on their transaction history and spending habits.
One of the key benefits of AI and ML in banking operations is the ability to reduce costs. By automating tasks, banks can reduce the need for manual labour and increase efficiency. This can lead to significant cost savings over time.
AI in Capital Markets and Investments
In capital markets and investments, AI and ML are used to provide real-time market data analysis and identify potential risks. These technologies are used to analyze large amounts of data and identify patterns that may not be visible to human analysts. This can help investors make more informed decisions and reduce their risk exposure.
AI and ML are also being used to automate trading strategies. These technologies can analyze market data and execute trades based on pre-defined rules and algorithms. This can help investors take advantage of market opportunities in real-time.
One of the key benefits of AI and ML in capital markets and investments is the ability to improve accuracy. By analyzing large amounts of data, these technologies can provide more accurate predictions and reduce the likelihood of errors.
In conclusion, AI and ML are becoming increasingly important in the banking and capital markets industry. These technologies improve operations, increase efficiency, and reduce costs. They are also used to enhance risk management and provide more accurate predictions. As these technologies continue to evolve, they will play an even more significant role in shaping the future of the banking and capital markets industry.
Challenges and Solutions in Implementing AI and Machine Learning
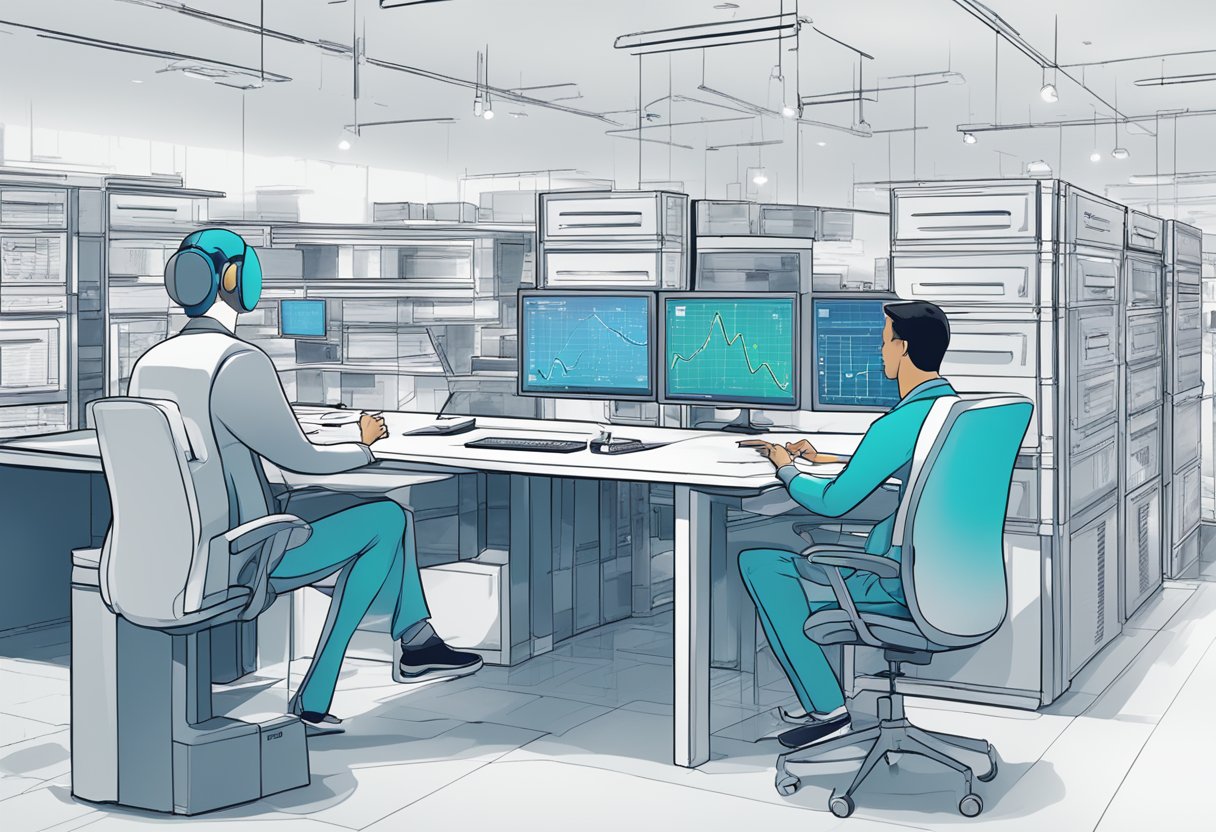
Addressing Model Risk and Bias
One of the main challenges in implementing AI and machine learning in post-trade risk assessment is addressing model risk and bias. Model risk is the risk of financial loss resulting from errors in developing, implementing, or using AI and machine learning models. BConversely, bias refers to the systematic errors that can occur in AI and machine learning models, resulting in inaccurate or unfair results.
To address model risk, financial institutions must establish robust model governance frameworks that ensure AI and machine learning models' accuracy, reliability, and integrity. This includes implementing effective model validation processes that assess the accuracy and reliability of models, as well as monitoring and reporting mechanisms that identify and address model errors and deficiencies.
To address bias, financial institutions need to implement measures that ensure the fairness and accuracy of AI and machine learning models. This includes establishing clear and transparent data governance policies that ensure the quality and integrity of structured and unstructured data used in models and implementing techniques such as explainable AI and model interpretability that enable users to understand how models arrive at their results.
Overcoming Data-Related Challenges
Another challenge in implementing AI and machine learning in post-trade risk assessment is overcoming data-related challenges. This includes the availability and quality of structured and unstructured data and the complexity of integrating and processing large volumes of data.
To overcome these challenges, financial institutions must establish effective data management and integration frameworks that ensure the availability, quality, and accuracy of structured and unstructured data in AI and machine learning models. This includes implementing data governance policies that ensure the integrity and security of data, as well as leveraging advanced data analytics techniques such as natural language processing (NLP) and machine learning algorithms that enable the processing and analysis of unstructured data.
In conclusion, while implementing AI and machine learning in post-trade risk assessment presents significant challenges, financial institutions can overcome these challenges by establishing robust model governance and data management frameworks that ensure models' accuracy, reliability, and fairness. Financial institutions can unlock the full potential of AI and machine learning in post-trade risk assessment by leveraging advanced data analytics techniques and implementing measures that ensure the quality and integrity of structured and unstructured data,
The Future of AI and Machine Learning in Post-Trade Risk Assessment
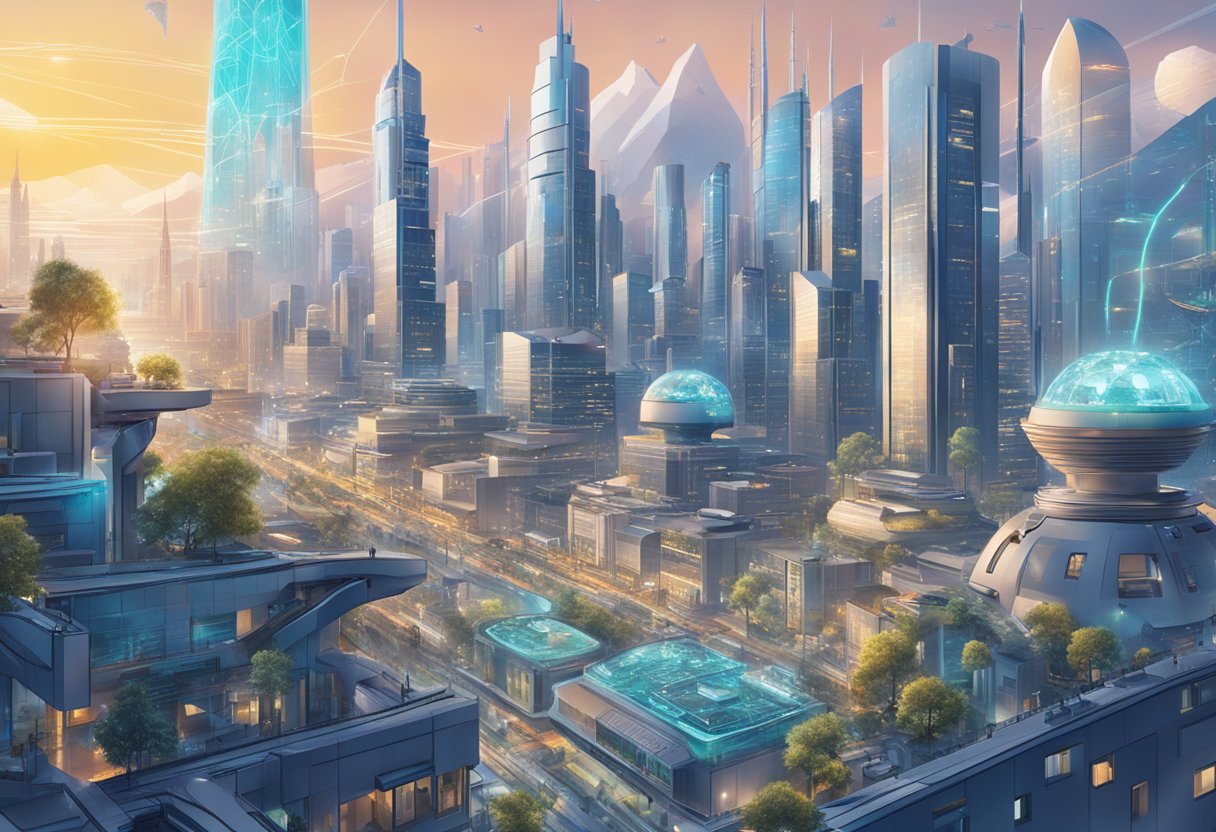
As the financial industry continues to evolve, the role of artificial intelligence (AI) and machine learning (ML) in post-trade risk assessment is becoming increasingly important. These technologies can revolutionize financial institutions' management of risk and decisions, resulting in more efficient and effective post-trade processing.
Predictive Analytics and Decision Making
One of the key benefits of AI and ML in post-trade risk assessment is the ability to leverage predictive analytics to make more informed decisions. By analyzing large amounts of data from a variety of sources, these technologies can identify patterns and trends that may not be immediately apparent to human analysts. This allows financial institutions to make more accurate predictions about future market conditions and adjust their risk management strategies accordingly.
In addition to improving decision-making, predictive analytics can help financial institutions identify potential risks before they materialize. By analyzing historical data and identifying patterns indicative of future problems, AI and ML can help institutions take proactive measures to mitigate risk and prevent losses.
Automation and Efficiency in Post-Trade Processes
Another key benefit of AI and ML in post-trade risk assessment is the ability to automate many processes involved in managing risk. This includes everything from data collection and analysis to risk modelling and reporting. By automating these processes, financial institutions can reduce the risk of human error and free up valuable resources that can be used to focus on more strategic initiatives.
In addition to improving efficiency, automation can help financial institutions stay ahead of the curve when managing risk. By analyzing large amounts of data in real-time, AI and ML can identify potential risks and alert institutions to potential problems before they materialize. This allows institutions to take proactive measures to mitigate risk and prevent losses, resulting in a more secure and stable financial system.
Overall, the future of AI and ML in post-trade risk assessment is bright. These technologies can potentially revolutionize how financial institutions manage risk and make decisions, resulting in a more efficient and effective post-trade processing system. By leveraging predictive analytics and automation, financial institutions can stay ahead of the curve when it comes to managing risk and ensure a more secure and stable financial system for years to come.