Credit Risk Modeling in Post-Trade Service Onboarding: A Comprehensive Guide
Effective credit risk modeling for post-trade onboarding. Our expert models ensure efficient trade processing and risk management. Learn more now.
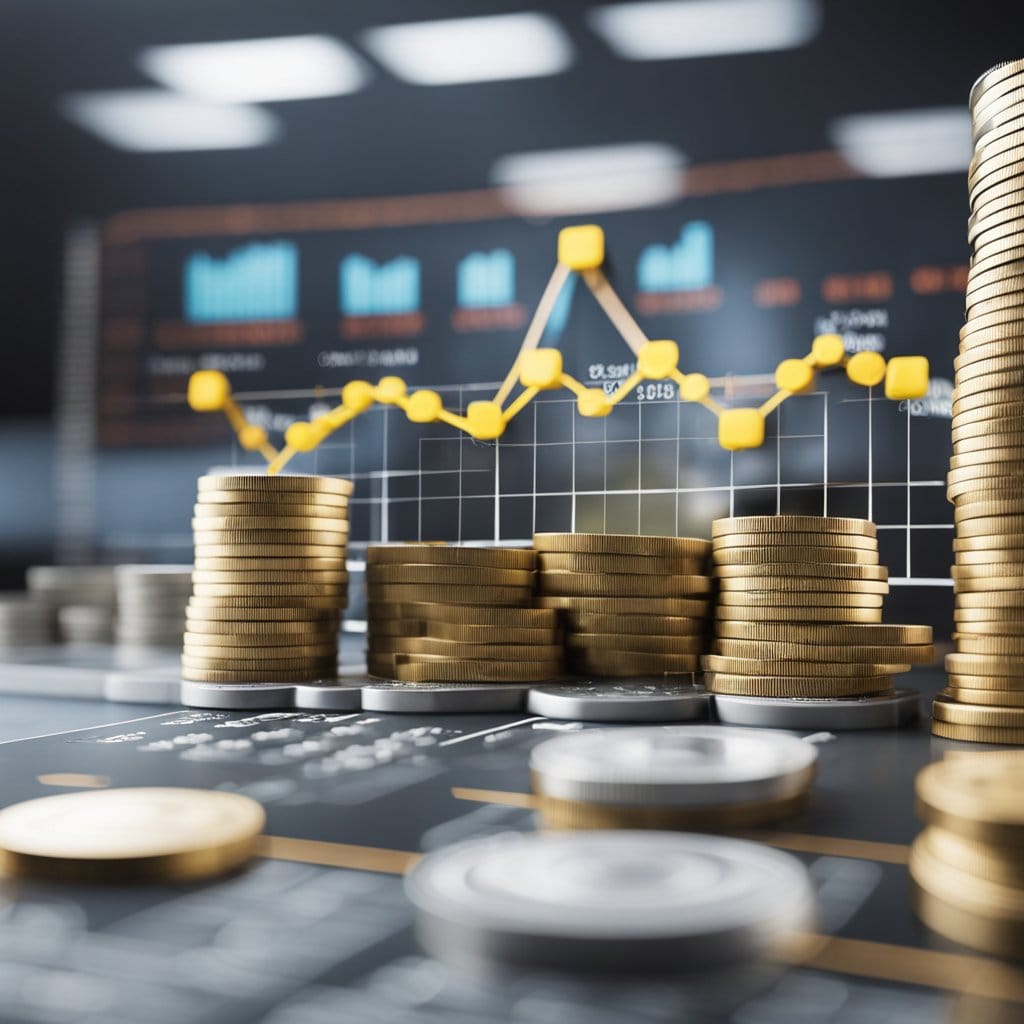
Credit risk modelling is essential to post-trade service onboarding in the financial sector. It involves using sophisticated analytical models to measure and manage credit risk associated with counterparties in the derivatives and securities markets. Credit risk modelling is complex and requires a deep understanding of trade processing and control mechanisms, risk management, and margin allocation.
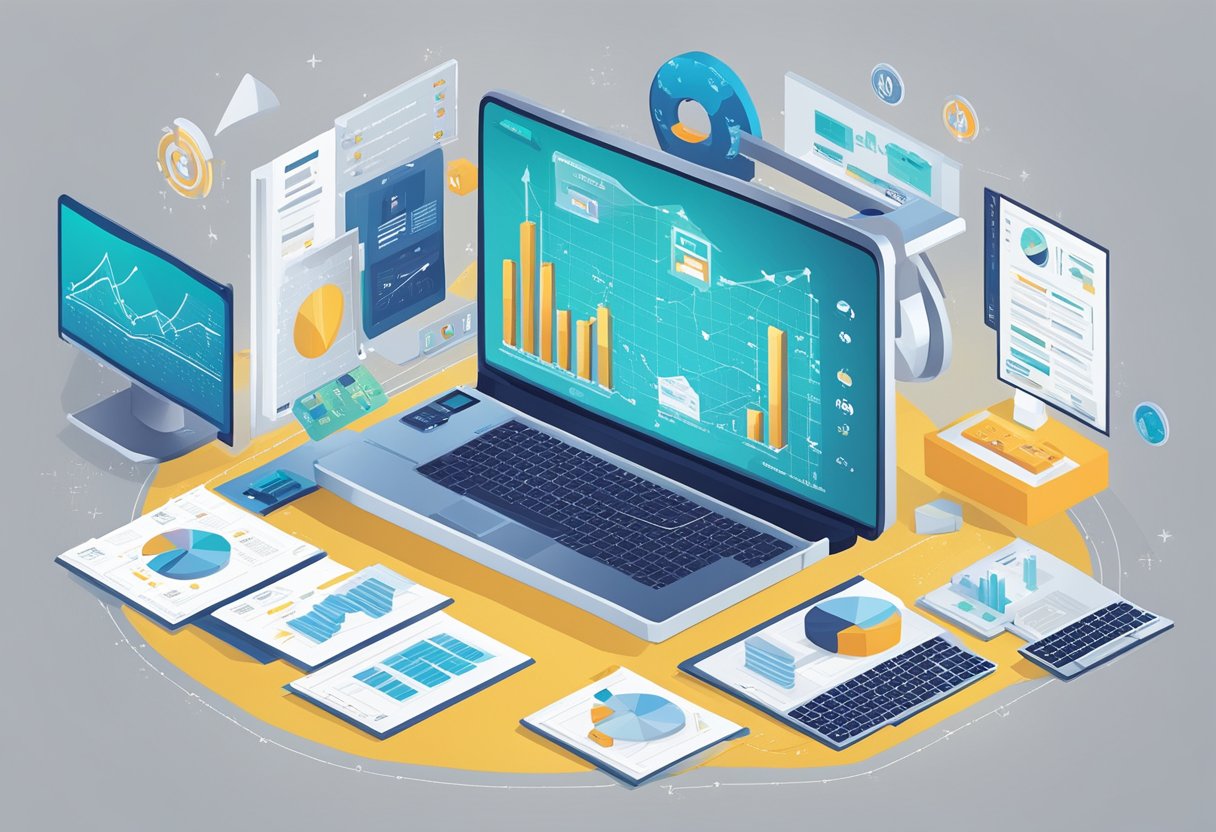
The role of technology in credit risk modelling has become increasingly important in recent years. Advanced technologies like machine learning and artificial intelligence have enabled financial institutions to analyze vast data and identify potential credit risks. As a result, credit risk modelling has become more accurate and efficient, enabling banks and other financial institutions to manage their risk exposure more effectively.
Key Takeaways
- Credit risk modelling is critical to post-trade service onboarding in the financial sector.
- Technology is crucial in credit risk modelling, enabling financial institutions to analyze vast data and identify potential credit risks.
- Credit risk modelling requires a deep understanding of trade processing and control mechanisms, risk management, and margin allocation.
Understanding Credit Risk
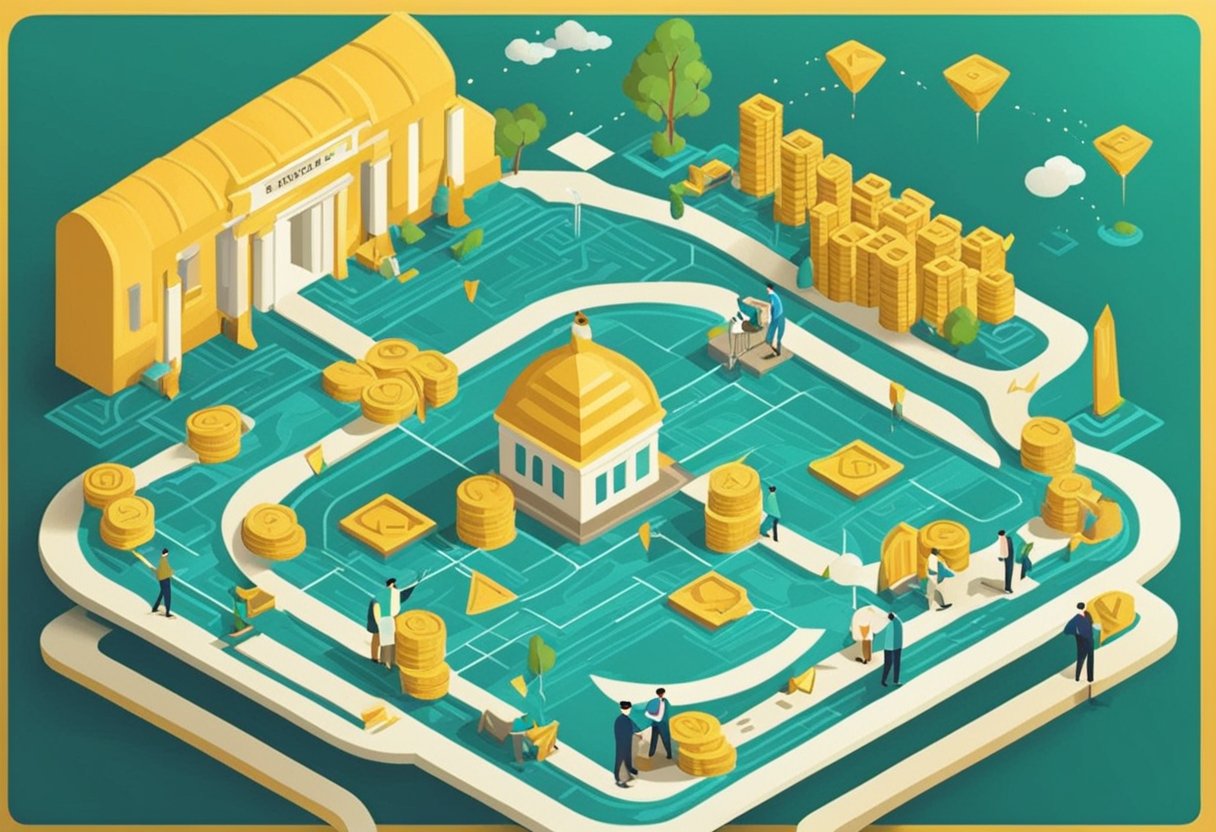
Credit risk is the risk of loss that a lender or investor incurs when a borrower fails to repay a loan or meet its contractual obligations. In post-trade service onboarding, credit risk is the risk that a counterparty will default on its obligations, leading to financial loss for the financial institution.
Credit assessment evaluates a borrower's creditworthiness, which involves analyzing the borrower's financial statements, credit history, and other relevant information. Credit analytics uses statistical models and data analysis techniques to assess credit risk and make informed lending decisions.
Probability of default (PD) is a critical metric in credit risk modelling, which is used to estimate the likelihood of a borrower defaulting on a loan or other credit obligation. PD models use a variety of factors, including financial ratios, credit history, and macroeconomic indicators, to estimate the probability of default.
PD models can be divided into two categories: structural models and reduced-form models. Structural models attempt to model the underlying economic factors that drive default risk. In contrast, reduced-form models use statistical techniques to estimate the probability of default without explicitly modelling the underlying economic factors.
PD models are often used with other credit risk modelling techniques, such as loss-given default (LGD) and exposure at default (EAD), to estimate the overall credit risk associated with a particular counterparty or portfolio of counterparties.
Overall, credit risk modelling is a critical component of post-trade service onboarding, as it helps financial institutions assess and manage the credit risk associated with their counterparties. Financial institutions can use sophisticated credit risk models and analytics to make informed lending decisions and minimize their exposure to credit risk.
Post-Trade Services in Financial Sectors
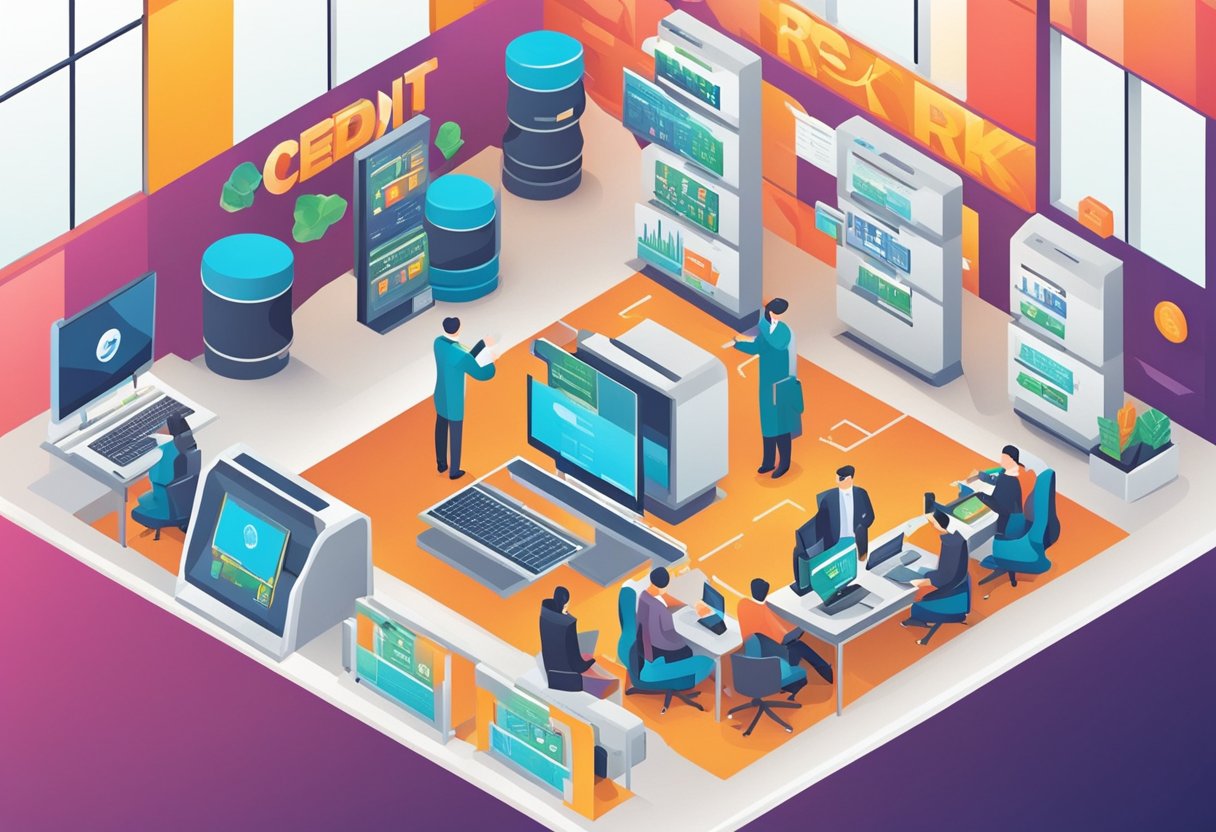
Post-trade services in financial sectors refer to the activities after a trade has been executed. These services include clearing, settlement, and custody of securities. Post-trade services ensure the transaction is completed successfully and the parties involved have fulfilled their obligations.
Post-trade services are critical to the smooth operation of financial markets. They help reduce counterparty risk, increase transparency, and ensure the financial system's integrity. Financial institutions like banks and broker-dealers rely on post-trade services to manage risk exposure and comply with regulatory requirements.
Clearing houses, also known as central counterparties (CCPs), play a crucial role in post-trade services. They act as intermediaries between buyers and sellers, guaranteeing the performance of each party to the transaction. CCPs also manage the risk associated with the transaction by requiring collateral from each party and monitoring the market for potential risks.
Exchanges also play a significant role in post-trade services. They provide a platform for trading securities and ensure the transaction is executed fairly and transparently. Exchanges also offer a price discovery mechanism, which helps ensure that the transaction is completed at a reasonable price.
In summary, post-trade services are essential to the smooth operation of financial markets. They help reduce counterparty risk, increase transparency, and ensure the financial system's integrity. Financial institutions, clearing houses, and exchanges all play a critical role in providing these services.
Role of Technology in Credit Risk Modeling
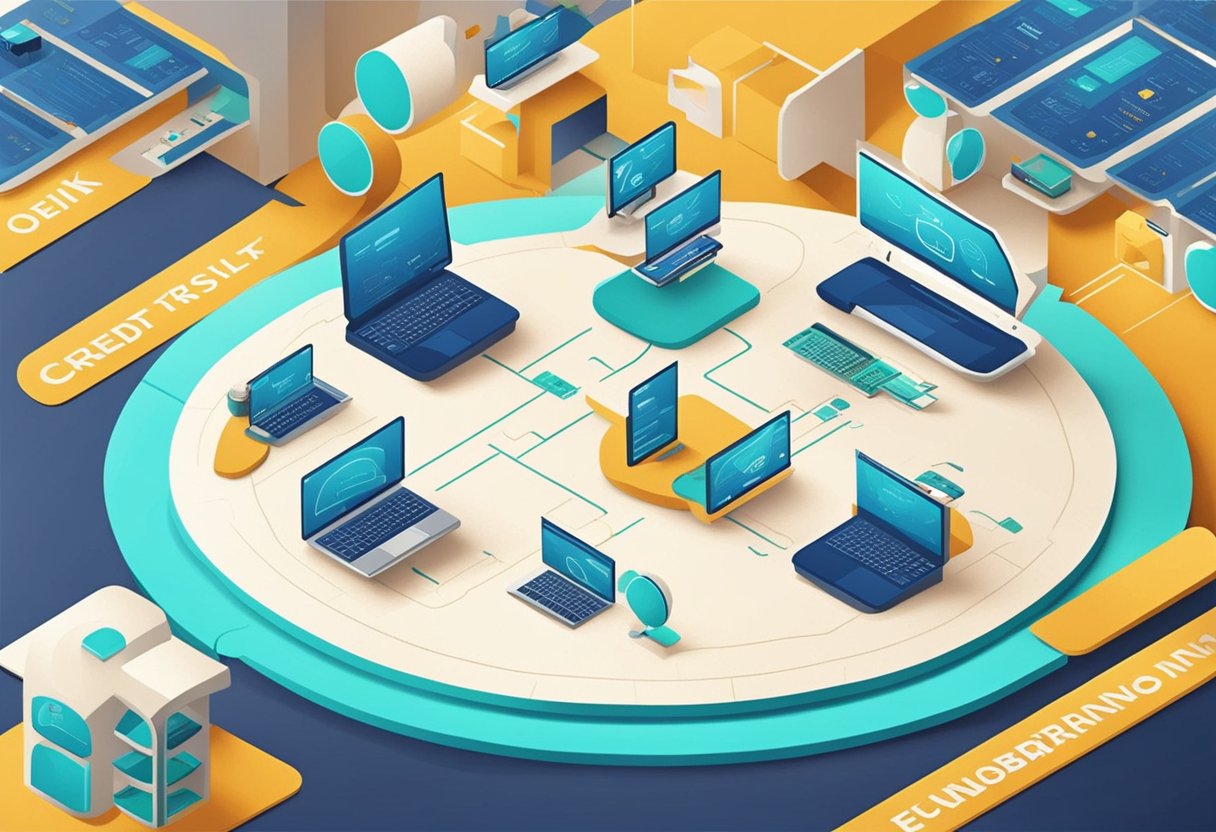
Technology plays a crucial role in credit risk modelling. In the past, credit risk models were developed using statistical models that relied on structured data inputs such as financial statements, credit scores, and payment histories. However, with the advent of digital transformation, the amount of unstructured data available has increased exponentially. This has led to the need for more sophisticated models that can handle both structured and unstructured data.
The use of technology in credit risk modelling has also led to the development of machine learning algorithms that can learn from historical data and make predictions based on that data. These algorithms can analyze large datasets and identify patterns that would be difficult for humans to detect. This has led to more accurate predictions of credit risk, which in turn has led to better risk management practices.
However, the use of technology in credit risk modelling also comes with its own set of challenges. One of the biggest challenges is model risk. Model risk refers to the risk that the model is used to make inaccurate or flawed predictions. This can lead to incorrect decisions, which can severely affect financial institutions.
To mitigate model risk, financial institutions need to ensure that their models are regularly tested and validated. They also need to ensure their models are transparent and explainable so stakeholders can understand how decisions are made.
In conclusion, the role of technology in credit risk modelling cannot be overstated. It has led to more accurate credit risk predictions and better risk management practices. However, financial institutions must be aware of the challenges of using technology in credit risk modelling and take steps to mitigate those risks.
Trade Processing and Control
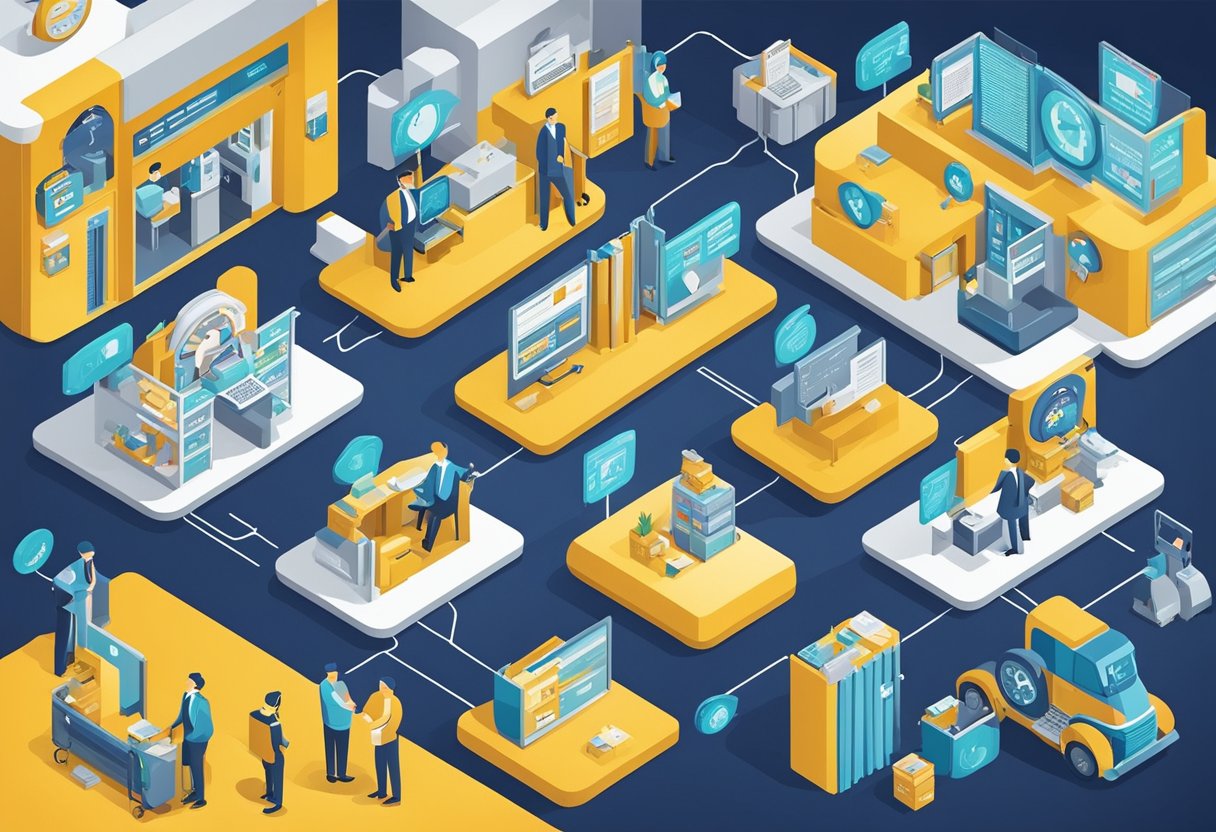
In the post-trade service onboarding process, trade processing and control are critical for ensuring transparency and reducing credit risk. Trade processing involves the confirmation and settlement of trades between counterparties. Control mechanisms are in place to manage the risk associated with these trades, including credit risk.
Real-time trade processing and confirmation enable counterparties to quickly agree on trade details, reducing the likelihood of errors and discrepancies. Electronic trade processing and confirmation platforms have become increasingly common, allowing faster and more efficient processing.
Control mechanisms are in place to ensure that trades are settled promptly and accurately. These mechanisms may include pre-trade checks, post-trade reconciliation, and margin requirements. By implementing these controls, counterparties can reduce the risk of credit losses and improve the transparency of the trade processing process.
Transparency is critical in the post-trade service onboarding process, as it allows counterparties to monitor trades' status and identify potential issues. Electronic trade processing and control platforms can increase transparency, as counterparties can access real-time trade status and settlement information.
In summary, trade processing and control are critical components of the post-trade service onboarding process. Real-time trade processing and confirmation, control mechanisms, and transparency are all essential factors in reducing credit risk and ensuring the efficient settlement of trades.
Risk Management and Margin Allocation
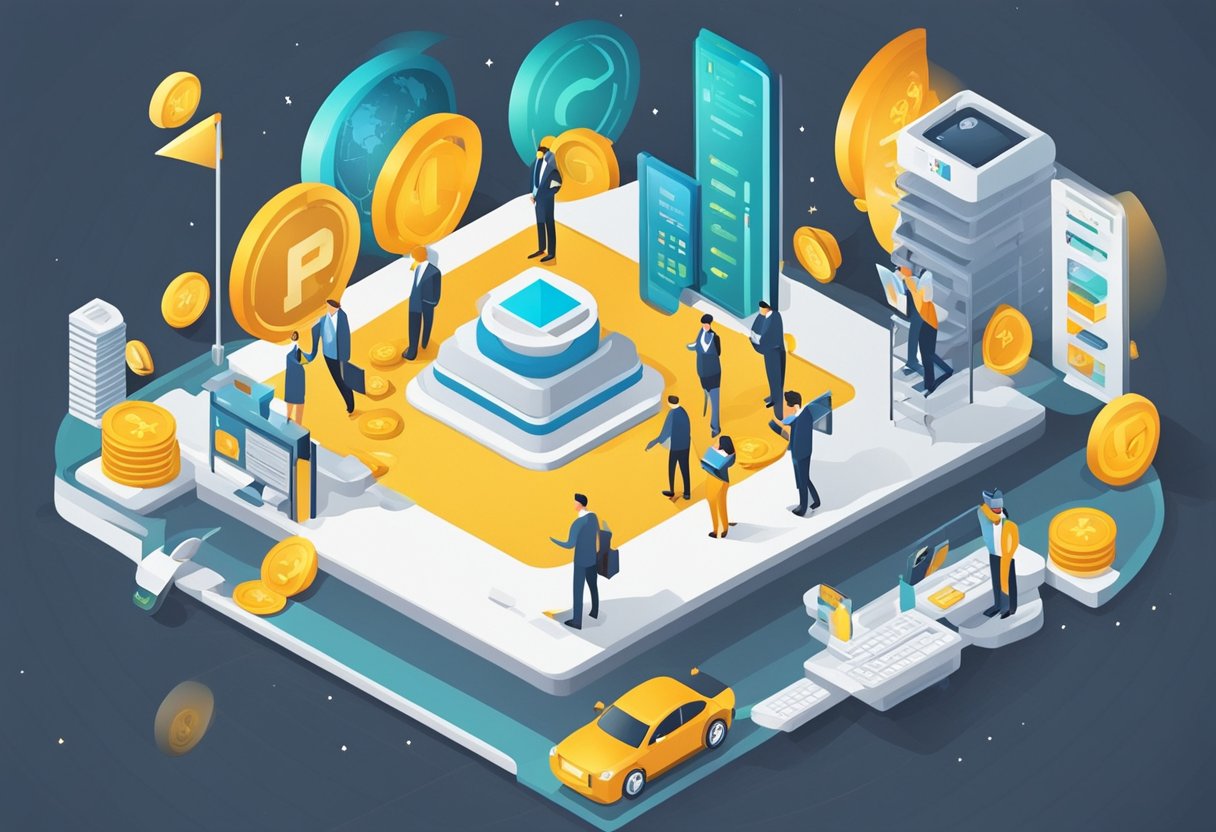
Risk management is crucial in ensuring the safety and stability of financial transactions in post-trade service onboarding. Credit risk modelling is used to assess the likelihood of default by borrowers and to estimate the potential loss in case of default. The process involves using advanced models to measure and manage credit risk, including calculating the initial margin.
The initial margin is a returnable deposit based on the open positions of a trader and any possible margin offsets. It acts as a buffer against potential losses and is calculated using sophisticated margin models. The ICE Risk Model, for example, is used at most clearing houses to calculate futures and options margin daily.
Margin allocation is another crucial aspect of risk management in post-trade service onboarding. It involves the allocation of margins among different types of trades or other traders. The process can be complex and requires careful consideration of various factors, including the risk profile of each trade and the firm's overall risk exposure.
Monitoring is also critical in risk management and margin allocation. It involves continuously monitoring trades and positions to ensure that they are within the limits set by the firm. The process may include using automated risk management systems that alert traders and risk managers in case of breaches.
Risk management and margin allocation are essential components of post-trade service onboarding. They help ensure the safety and stability of financial transactions by assessing and managing credit risk, calculating initial margin, allocating margin among trades and traders, and monitoring trades and positions.
Counterparty Credit Risk in Derivatives and Securities
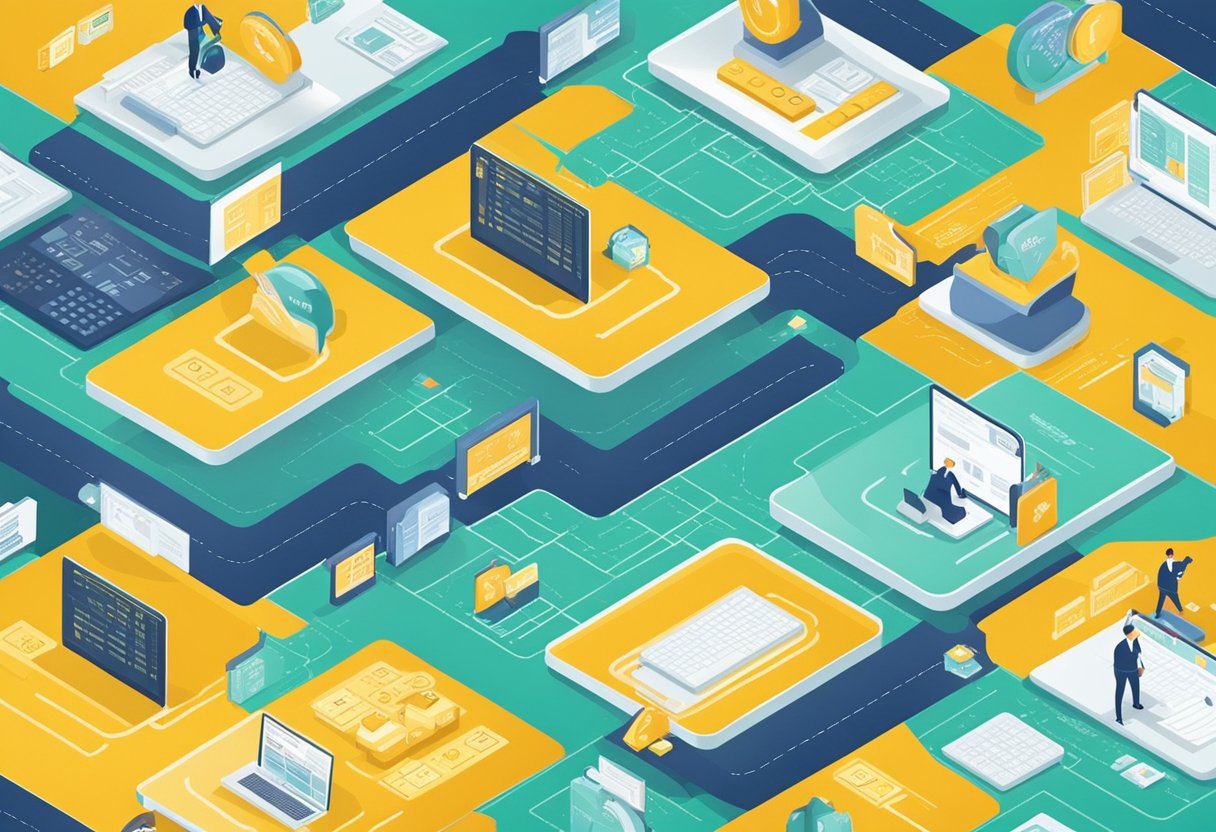
Counterparty credit risk (CCR) is the risk that a counterparty to a financial transaction will default before the final settlement of the transaction. In the derivatives and securities markets, CCR is a significant concern due to the high leverage involved in these instruments.
Derivatives are financial instruments whose value is derived from an underlying asset or index. They include options, futures, and other contracts. Options give the holder the right, but not the obligation, to buy or sell an underlying asset at a predetermined price and time. Futures are contracts to buy or sell an asset at a future date and price.
Securities are financial instruments representing ownership in a company or a debt obligation. They include stocks, bonds, and other debt securities.
Counterparty credit risk in derivatives and securities arises from the potential for losses due to the default of a counterparty. For example, if a counterparty defaults on a derivatives contract, the other party may suffer a significant loss.
Market participants use various techniques to manage counterparty credit risk, including netting, collateral, and credit limits. Netting involves offsetting exposures between two counterparties to reduce the overall credit risk. Collateral is an asset or cash pledged by a counterparty to secure a transaction. Credit limits are used to limit the amount of exposure to a counterparty.
Counterparty credit risk varies across different asset classes and positions. For example, credit risk in equity derivatives is typically lower than in credit derivatives due to the higher liquidity of the underlying assets. Similarly, credit risk in collateralized debt obligations (CDOs) is higher than in other securities due to the complexity of the underlying assets.
Overall, effective counterparty credit risk management is critical to the stability and efficiency of the derivatives and securities markets. Market participants need robust risk management frameworks to identify, measure, monitor, and control counterparty credit risk.
Impact of Accounting Standards and Regulatory Approaches
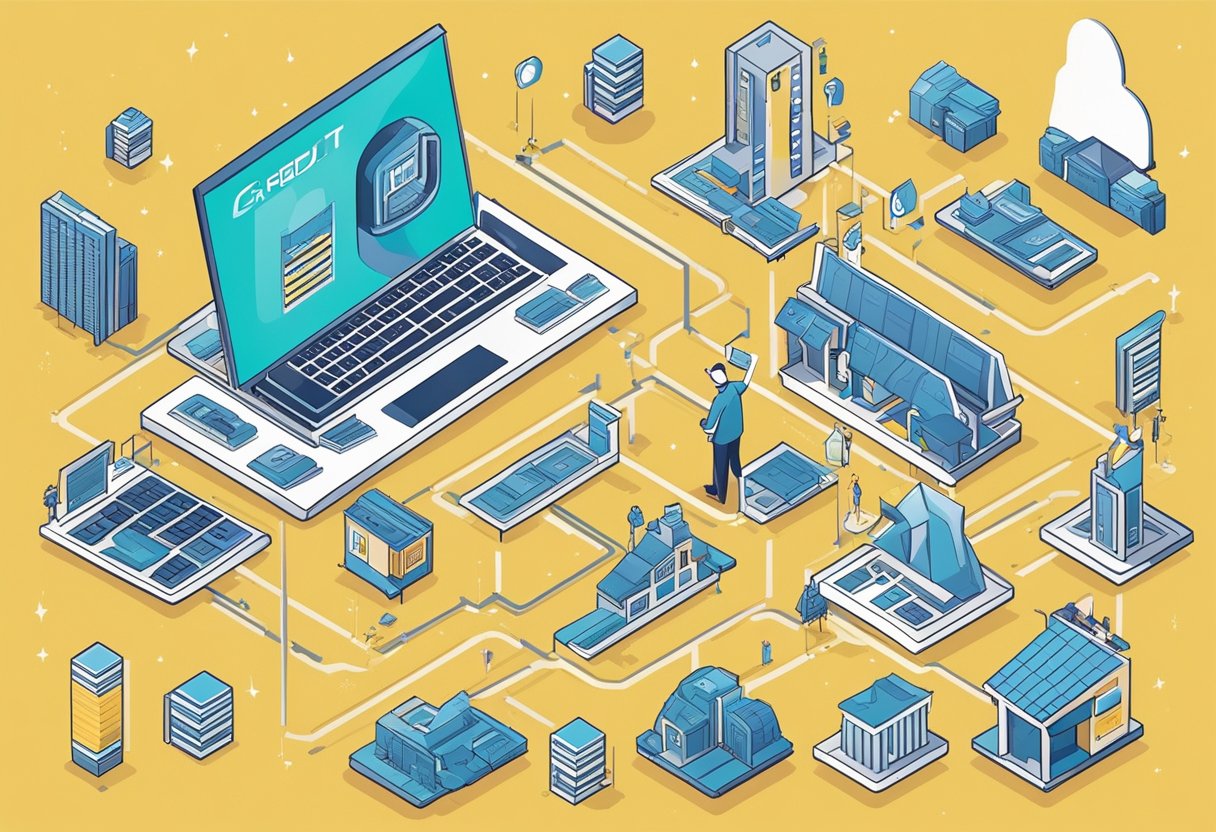
The implementation of accounting standards and regulatory approaches has a significant impact on credit risk modelling in post-trade service onboarding. Adopting the current expected credit loss (CECL) accounting standard, which replaces the incurred loss model, requires institutions to make changes across credit risk modelling, risk tolerance, capital planning, and financial reporting. According to McKinsey & Company [1], US banks have been preparing for the implementation of CECL, which took effect on December 15, 2019.
In addition to accounting standards, regulatory approaches such as the standardized approach (SA) and internal ratings-based (IRB) approach also affect credit risk modelling. The Basel Committee on Banking Supervision [2] provides a comprehensive report on credit risk modelling practices and applications, covering the objectives, methodologies, data requirements, validation, and regulatory issues of credit risk modelling.
The SA is a regulatory approach that requires banks to hold capital based on standardized risk weights for different asset classes. On the other hand, the IRB approach allows banks to use their internal models to estimate risk parameters such as probability of default, loss given default, and exposure at default. The use of advanced credit risk modelling and the regulatory framework under the IRB approach is examined by Oberson and Dong [3], who also investigate whether banks' opportunistic behaviour drives the adoption choice of the Capital Treatment Approach.
Furthermore, accounting standards and regulatory approaches are also considered in credit risk modelling by S&P Capital IQ [4]. Their credit risk models provide a range of credit risk measures, including expected default frequency, loss-given default, and credit risk scores, which are used for credit analysis and stress testing.
Overall, the impact of accounting standards and regulatory approaches on credit risk modelling in post-trade service onboarding is significant. Institutions must consider these factors when developing and implementing credit risk models to ensure compliance with regulations and accurate financial reporting.
[1] McKinsey & Company. (2019, July 15). The strategic implications of CECL. https://www.mckinsey.com/industries/financial-services/our-insights/banking-matters/the-strategic-implications-of-cecl
[2] Basel Committee on Banking Supervision. (2005, May). Credit risk modelling: Current practices and applications. https://www.bis.org/publ/bcbs49.pdf
[3] Oberson, R., & Dong, Y. (2020). Moving toward the Expected Credit Loss Model under IFRS 9: Capital Treatment Approach Adoption and Opportunistic Behavior. https://www.ifrs.org/content/dam/ifrs/events-and-conferences/2020/iasb-research-forum/4-oberson-dong.pdf
[4] S&P Capital IQ. (n.d.). Credit risk models. https://www.capitaliq.com/products/credit-risk-models.html
Role of Different Entities in Credit Risk Modeling
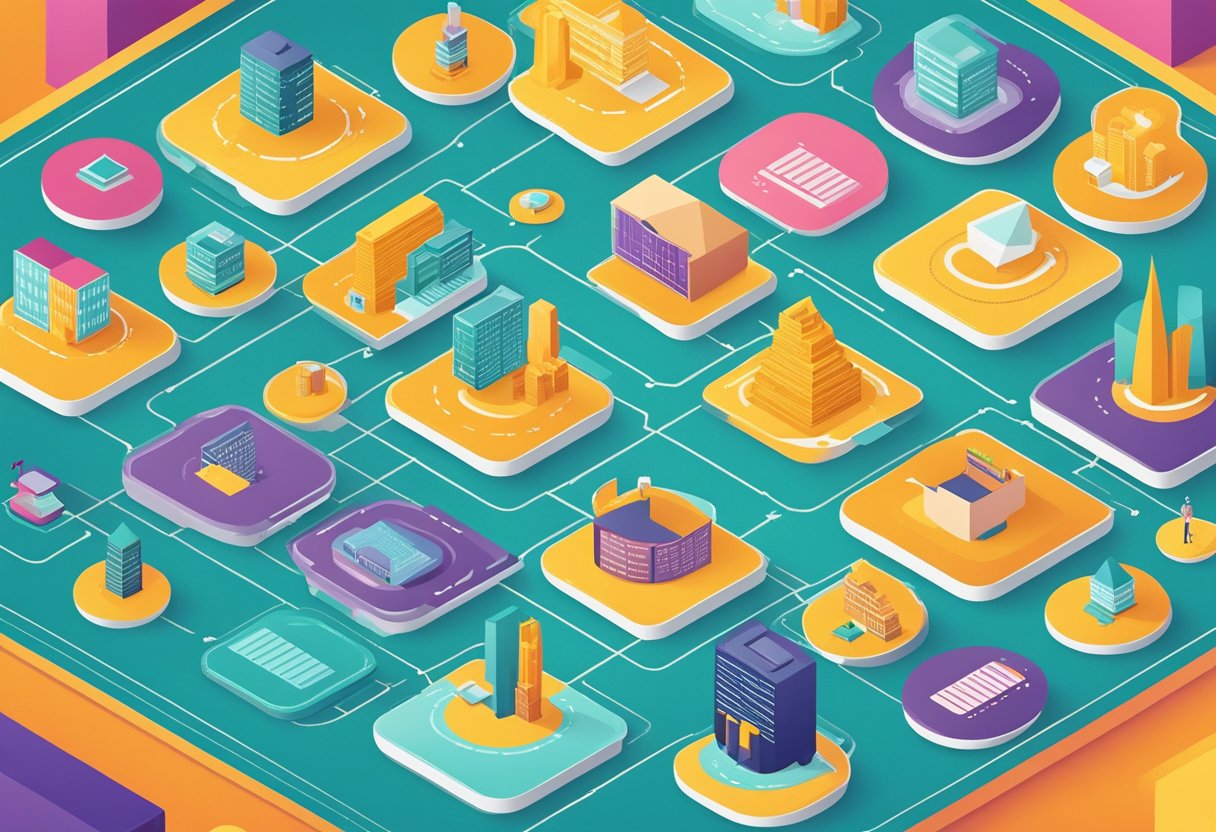
Credit risk modelling involves multiple entities, each with a unique role. Investment managers play a crucial role in credit risk modelling, as they are responsible for making investment decisions based on the creditworthiness of potential clients. They use various tools and techniques to assess credit risk, such as analyzing company financial data, insurance, and consumer credit reports.
Regulatory bodies such as the Securities and Exchange Commission (SEC) are essential in credit risk modelling. They set guidelines and regulations that investment managers must follow when assessing credit risk. For example, the SEC requires investment managers to disclose their credit risk modelling methodologies and report any changes to those methodologies.
The country and industry risk scores are also important factors in credit risk modelling. These scores help investment managers assess the creditworthiness of potential clients based on the economic and political conditions of the country or industry in which they operate. Financial ratios, such as debt-to-equity and interest coverage ratios, are also commonly used in credit risk modelling.
Receiver operating characteristic (ROC) analysis is another essential tool in credit risk modelling. ROC analysis helps investment managers determine the accuracy of their credit risk models by measuring the trade-off between sensitivity and specificity. This analysis is crucial in ensuring investment managers make informed investment decisions based on accurate credit risk assessments.
Finally, customer experience is an essential factor in credit risk modelling. Investment managers must ensure that their credit risk models are user-friendly and provide accurate and timely information to clients. This helps build trust and confidence in the investment management process, which leads to increased customer satisfaction and loyalty.