Data Lakes in Post-Trade Data Management: Efficient Solutions for Modern Finance
Discover how data lakes can revolutionize post-trade data management in modern finance. Read our informative article for efficient solutions today.

The rapid growth of technology in the financial sector has led to an influx of data that organizations must manage and analyze. Data lakes have emerged as a powerful solution for handling vast amounts of raw data, offering storage and processing capabilities in a central repository. In post-trade data management, data lakes play a pivotal role in streamlining the processes involved in settlement, payment, and custody while maintaining regulatory compliance and ensuring data security.
As the middle office in financial institutions often subscribes to many data sources to perform risk calculations, post-trade systems need to utilize this information effectively. Modernizing post-trade technology with data lake implementations allows firms to enhance their data management practices, improve operational efficiency, and enable more informed decision-making in an increasingly competitive industry.
Key Takeaways
- Data lakes are central repositories for storing and processing large volumes of raw data in the financial sector.
- Effective utilization of data lakes in post-trade data management can improve operational efficiency and decision-making.
- Adopting data lake technology enables firms to maintain regulatory compliance and ensure data security in trading processes.
Understanding Data Lakes and Post-Trade Data Management
Data lakes play a crucial role in the modern world of data management, serving as a centralized repository for storing vast amounts of raw and unprocessed data from various sources. In post-trade data management, data lakes provide an essential foundation for handling the massive amount of data generated during the post-trade processing lifecycle.
Post-trade data management involves processing, validating, and reconciling trade data using various services and technology systems. Data lakes offer a flexible and scalable data storage solution that enables financial institutions to efficiently store, process, and analyze the diverse data types associated with post-trade activities.
Using data lakes for post-trade data management helps organizations effectively manage their data from multiple trading platforms, custodian banks, and other external data sources. By consolidating all this data into a single repository, financial institutions can generate insights, identify trends, and address compliance requirements more efficiently.
Moreover, the flexibility of data lakes allows institutions to quickly adapt to changes in the financial industry, such as new regulations or the introduction of new financial products. With the ability to store and process data at a granular level, organizations can gain valuable insights from their post-trade data, enabling them to optimize their operations and mitigate risks associated with trade settlements.
In summary, data lakes are instrumental in simplifying and improving post-trade data management, offering a scalable and flexible solution for organizations to efficiently handle the large volumes of data generated during post-trade processing. By consolidating and processing all relevant trade data in a central location, financial institutions can drive better decision-making and meet the evolving demands of the industry.
Impact of Technology on Post-Trade Processes
The evolution of technology has brought significant advancements to the world of post-trade data management. With the increasing complexity of financial markets, traditional methods of handling trade data have become inadequate, prompting market participants to embrace new solutions. In this context, data lakes have emerged as an innovative approach to manage and analyze trade data efficiently.
One of the significant technological developments affecting post-trade processes is the widespread adoption of APIs. These interfaces allow various systems to communicate and exchange information seamlessly. With APIs, post-trade systems can be integrated with other trading platforms, risk management systems, and regulatory reporting solutions, streamlining the overall process and reducing manual interventions.
Another technology reshaping post-trade data management is the use of web browsers as a means to access trade data. Advanced browser-based systems enable real-time access to trade information, allowing multiple users to access and analyze data without specialized software. This flexibility not only simplifies data accessibility but also supports collaboration across different teams, improving decision-making and overall efficiency.
As the volume of trade data continues to grow, the implementation of data lakes presents a scalable solution to store and process vast amounts of diverse data. Data lakes enable organizations to store structured and unstructured data in their native formats, allowing for more in-depth and flexible analytics. This consolidation of data sources simplifies data management while enabling more granular insights into trading activity, risk exposure, and regulatory compliance.
Moreover, the application of machine learning and artificial intelligence (AI) is revolutionizing how post-trade data is analyzed. AI algorithms are capable of processing vast amounts of trade data quickly and identifying patterns, trends, and anomalies that would otherwise remain hidden. This advanced data analytics capability enables market participants to uncover new opportunities and risks, optimize post-trade processes, and strengthen regulatory compliance efforts.
In conclusion, technology is transforming post-trade data management across the financial industry. The adoption of APIs, browser-based systems, data lakes, and AI-driven analytics is driving increased efficiency, collaboration, and strategic decision-making, ensuring market participants can better navigate the complexities of today's rapidly evolving trading landscape.
Data Management and Security in Trading
Data lakes play a crucial role in post-trade data management due to their capacity to store vast amounts of data in their native formats. These large, centralized repositories can efficiently manage various data types, such as trade details, financial market data, and regulatory compliance information 1. Consequently, organizations dealing with trading activities increasingly employ data lakes to harness the power of machine learning and analytics to extract valuable insights.
Data Lake Management is essential to optimize the usage of these repositories and ensure seamless data retrieval and processing. However, managing data lakes can be challenging due to various formats, lack of metadata, and autonomous data changes 2. Organizations must implement robust metadata management practices, address data quality issues, and focus on data curation and cataloguing to streamline operations.
Security is a crucial aspect of data lake management in the trading context. Unauthorized access to sensitive financial data could result in misuse, leading to severe consequences 3. Therefore, implementing robust security measures, such as access controls and encryption, is vital 4.
Secure Access Control Systems prevent unauthorized users from accessing sensitive data within the data lake. Access control mechanisms like role-based access control (RBAC) or attribute-based access control (ABAC) can help implement granular access policies based on the user's role or attributes 5.
Data Encryption is another essential security aspect in trading data lakes. Financial data should be encrypted both at rest and in transit to ensure that even if unauthorized access occurs, the data would be unusable to the intruder 6.
In conclusion, efficient data management and security are critical for trading organizations leveraging data lakes for post-trade data management. Organizations must prioritize implementing robust access control mechanisms and encryption techniques to safeguard sensitive trading data and maintain their competitive edge in the market.
Architecture and Management of Data Lakes
Data lakes play a crucial role in post-trade data management, offering an effective solution for storing and processing large volumes of raw data collected from various sources. Their flexible and scalable architecture allows organizations to ingest and store data in multiple formats, unlike traditional data warehouses that usually require a predefined structure.
The architecture of data lakes is designed to handle the diverse needs of data storage and processing. Data is stored in its raw format and can be in structured, semi-structured, or unstructured formats. This flexibility allows organizations to bring different data types into the data lake, providing a single access point for analytics and reporting. The data lake should also include metadata management solutions, as metadata is essential for successfully organizing, searching, and accessing data within the data lake environment 1.
Management of data lakes involves maintaining data quality, ensuring data security, and establishing data governance policies. One of the challenges in data lake management is to balance the trade-offs between structure and flexibility. Some data lakes may lean towards a more structured approach, similar to data warehouses, while others may prefer the agility associated with flexible storage formats 2.
Data lake architectures can be compared to traditional data warehouses regarding their functionality and purpose. Both systems aim at integrating heterogeneous data from different sources into a single, homogeneous data management system, thereby overcoming the limits of disparate and isolated data silos. However, the key difference lies in their approach to data storage and processing. Data warehouses usually enforce a predefined schema, while data lakes support a schema-on-read approach, which promotes greater flexibility and adaptability 3.
In conclusion, the architecture and management of data lakes play a significant role in post-trade data management. With the right approach and proper maintenance, data lakes can provide valuable insights and help organizations make better-informed decisions.
Structuring and Analysis of Trading Data
In the world of post-trade data management, dealing with vast amounts of trading data is a crucial task. This data can be classified into two categories: structured data and unstructured data. Structured data comprises organized information that is easily searchable, such as trade dates, volumes, and prices. On the other hand, unstructured data encompasses text, images, and unorganized files that are hard to interpret and analyze without proper processing.
To leverage the benefits of this data, organizations can employ analytics and visualization tools. These analytical tools can efficiently manage, manipulate, and interpret large datasets, providing valuable insights for optimizing post-trade processes. Visualization tools are integral in presenting complex data comprehensibly, enabling easier analysis and informed decision-making.
Incorporating artificial intelligence (AI) into post-trade processing systems can further enhance the analysis of structured and unstructured data. AI-driven algorithms can automate data processing tasks, reducing manual intervention and improving overall efficiency. Additionally, AI can help organizations adapt to ever-changing market dynamics and regulatory frameworks by constantly analyzing data trends and discrepancies.
A key component in managing this vast amount of trading information is a data lake. A data lake is an extensive storage system that handles any data format, providing a unified space for storing and processing structured and unstructured data. By creating a consolidated view of trading data, data lakes enable organizations to streamline their post-trade processes better and achieve increased operational efficiency.
In conclusion, structuring and analyzing trading data is critical for efficient post-trade data management. By utilizing structured and unstructured data, harnessing the power of analytical and visualization tools, and incorporating AI into processing systems, companies can optimize their post-trade processes and achieve a competitive edge in the dynamic financial landscape.
Regulations and Compliance in Data Management
Data management practices, especially post-trade data management, are subject to numerous regulations and compliance requirements. These regulations aim to ensure the confidentiality, integrity, and availability of data, as well as protect the privacy of individuals involved in financial transactions.
One such regulatory standard is the General Data Protection Regulation (GDPR), which focuses on protecting personal data within the European Union. This regulation has a significant impact on data management processes as it mandates strict controls over the storage, access, and processing of personal data. Organizations must adopt measures to ensure compliance with the GDPR, such as implementing data protection policies and maintaining appropriate records of data processing activities.
Another regulation that affects data management is the California Consumer Privacy Act (CCPA). Similar to the GDPR, the CCPA strives to protect the privacy of consumers by imposing stringent rules on how businesses collect, store, and use their personal information. To comply with this regulation, organizations need to be transparent about their data collection practices and provide users with options to control the use of their data.
Meeting regulatory compliance in data management involves implementing robust data governance frameworks and adhering to established processes. These processes encompass various aspects, such as data quality, lifecycle management, and privacy controls. Implementing data lake governance, for instance, is vital to ensure that stored data is accurate, relevant, and securely accessible, as well as compliant with GDPR or CCPA requirements 1().
Moreover, reporting plays a crucial role in post-trade data management. Regulated entities must report transactional data according to specific formats and submission deadlines. Implementing technologies and processes that facilitate accurate and timely reporting is essential to meeting regulatory requirements.
In summary, adhering to regulations and maintaining compliance in post-trade data management is a complex but essential aspect of the financial industry. Organizations must continuously evaluate and adapt their data management practices to meet the ever-evolving regulatory landscape and protect the interests of their customers and stakeholders.
Technologies and Tools for Efficient Data Management
In post-trade data management, various technologies and tools are pivotal in ensuring efficient data storage, processing, and analysis. These tools help businesses handle vast amounts of structured and unstructured data, providing a seamless and well-organized environment for data-driven decision-making.
One of the primary components of data management is storage. Cloud technology has revolutionized how businesses store data, offering scalable solutions for growing data volumes. Services such as Amazon S3 are excellent storage options thanks to their reliability, durability, and cost-effectiveness. Cloud providers like AWS and Google Cloud also incorporate intuitive user interfaces that simplify data management tasks.
Extract, Transform, and Load (ETL) tools are critical for processing and preparing data for analysis. These tools can extract data from various sources, transform it into the desired format, and load it into a data warehouse. ETL solutions can handle structured and unstructured data formats such as JSON, Avro, and XML, ensuring versatility in data integration.
In addition to ETL tools, modern data management platforms rely on data warehousing solutions to perform advanced analytics on data. Amazon Redshift is a popular cloud-based data warehouse that offers fast query performance and flexibility. With broad support for standard SQL, Redshift enables users to perform updates, deletions, transactions, and analytical functions on data, driving better insights and decision-making processes.
Efficiency is key when dealing with massive amounts of post-trade data. By leveraging cloud-based storage and robust ETL tools, businesses can streamline their data management processes and obtain valuable insights from the data they have captured. Overall, these technologies and tools form the cornerstone for modern, efficient post-trade data management.
Use Cases and the Future of Post-Trade Data Management
Data lakes are becoming an integral part of post-trade data management in financial markets. They offer a flexible and scalable solution for storing massive amounts of data, allowing organizations to process and analyze data from various sources efficiently and in different formats. In this section, we will explore some use cases and the prospects of applying data lakes in post-trade data management.
One critical use case for data lakes in post-trade processing is risk management. Financial institutions need to assess and manage various types of risks, such as market risk, credit risk, and operational risk. Data lakes enable organizations to store and analyze large volumes of granular data, including trade and market data, and support complex analytical queries that provide critical insights into risk exposure. This comprehensive view of risk factors can help organizations better manage their risks and reduce potential losses.
Another use case for data lakes in post-trade data management is the development of new financial products. To stay competitive, financial institutions need to innovate and create new products that cater to the needs of their clients. Through analyzing patterns and trends in the data, organizations can identify potential opportunities and develop new products that meet market demands. Data lakes facilitate this process by providing a flexible and efficient platform for data analysis.
In addition to the above use cases, data lakes offer significant potential for leveraging machine learning techniques to optimize post-trade processing. Machine learning algorithms can help enhance various aspects of post-trade processing, such as automating reconciliation, detecting anomalies, and predicting potential issues before they arise. By harnessing the power of machine learning, organizations can improve the efficiency and accuracy of their post-trade processes, mitigate risks, and reduce costs.
In conclusion, data lakes are increasingly vital in post-trade data management, with use cases spanning risk management, new product development, and machine learning applications. As financial markets continue to evolve and generate ever-growing amounts of data, the adoption and importance of data lakes in post-trade data management are expected to grow, enabling more efficient and insightful decision-making processes for financial institutions.
In-depth Understanding of Unique Trading Terms
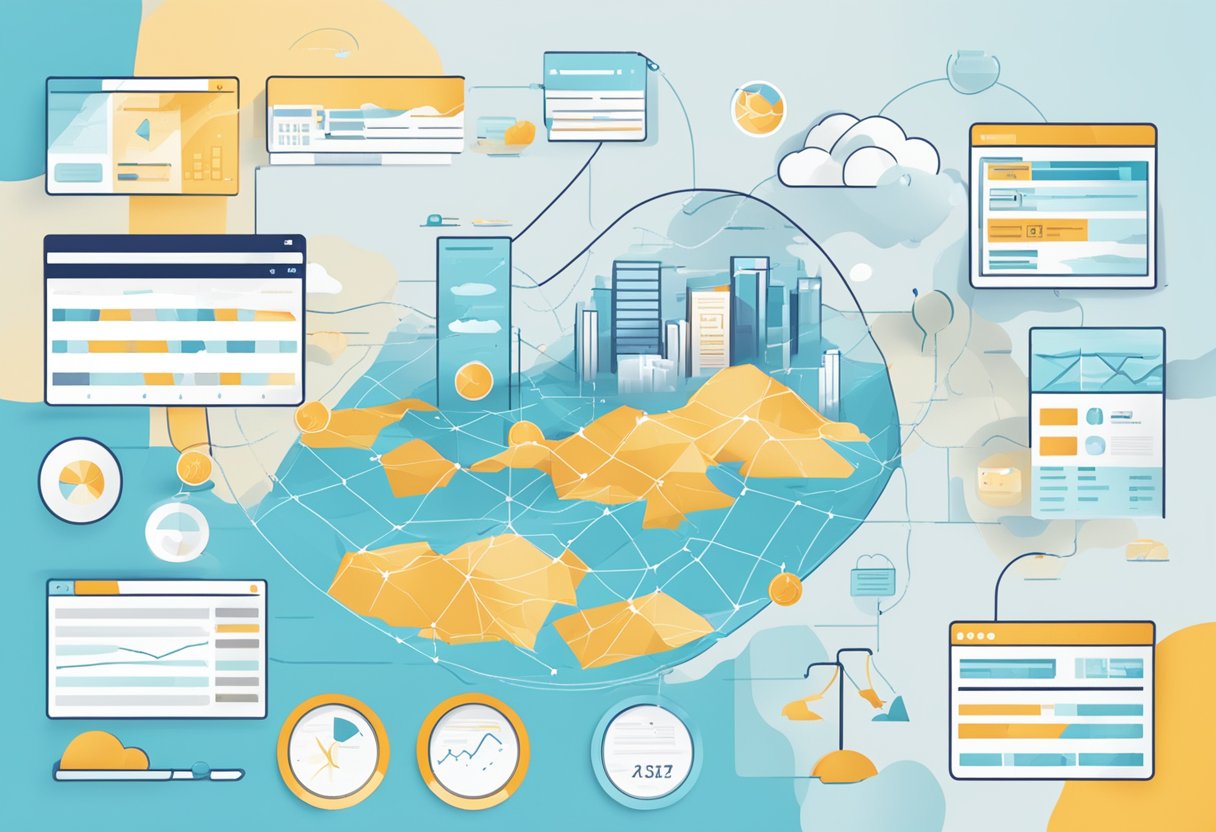
Post-trade data management encompasses a vast range of unique trading terms and concepts, which are essential to have a comprehensive understanding of the entire process. One such term is the GPTM or Global Post-Trade Modeling system. Gptm is a sophisticated model designed for seamless integration with various post-trade platforms, allowing for efficient analysis and optimization of trade-related data.
Semaphore Model Management is another vital component in post-trade data management. It is an advanced system that allows users to create, modify, and manage models for different trading scenarios. Semaphore Model Management enables traders and firms to develop precise approaches to address various trading challenges, facilitate the adoption of best practices, and ultimately improve overall performance.
A central aspect of post-trade data management is building a knowledge graph. This graph comprises a network of interconnected entities, properties, and relationships within the trading ecosystem, enabling a holistic understanding of the complex data landscape. Knowledge graphs provide a unified framework for the organization and retrieval of information, promoting efficient decision-making processes.
The next crucial element is ontology. In post-trade data management, ontology refers to the explicit and formal description of entities, properties, relationships, and categories within the trading domain. This comprehensive classification helps data lakes capture a clear semantic understanding of the collected data, ensuring structured data storage and retrieval, thereby facilitating better analysis.
Lastly, metadata plays an essential role in post-trade data management. Metadata refers to the descriptive information about the data collected in data lakes, such as source, context, format, and purpose. Effective management of metadata enriches the quality of the data lake, improves data integration, and ensures that stakeholders have quick access to accurate and relevant information.
In conclusion, a deep understanding of unique trading terms, semaphore model management, knowledge graph, ontology, and metadata is vital for efficient post-trade data management. These entities enable stakeholders to create a robust, structured, and unified framework to improve the analysis and decision-making process within the trading domain.